HSC 4500 RU Health & Medical Epidemiological Data Essay
ANSWER
Let’s analyze the provided data and answer the questions based on the information given:
Harris County
- Population at risk: 22,237,304
- Cases: 77,228
- Crude rate: 347.3
- Age-adjusted rate: 400.1
- 95% CI: 397.2 to 403.1
Bell County
- Population at risk: 1,659,684
- Cases: 6,733
- Crude rate: 405.7
- Age-adjusted rate: 480.8
- 95% CI: 469.1 to 492.6
Foard County
- Population at risk: 6,315
- Cases: 42
- Crude Rate: 665.1
- Age-adjusted rate: 404.9
- 95% CI: 280.4 to 573.7
Interpretation:
- Reliability of Data: The reliability of the data can be assessed based on the population size and the robustness of the rates provided. In this case, Harris County has the largest population at risk, which implies that the data for this county is likely to be more reliable due to the larger sample size. Larger sample sizes generally lead to more accurate and stable rate calculations. Bell County has a relatively moderate population size, and Foard County has the smallest population, making the data for these counties potentially less reliable due to the smaller sample sizes.
- Preventative Intervention: Foard County seems to require the most preventative intervention when it comes to cancer incidence rates. The crude rate for Foard County is significantly higher than that of Harris and Bell counties, indicating a higher number of cancer cases relative to the population. While the age-adjusted rate for Foard County is lower than its crude rate, it is still considerably higher than the rates for Harris and Bell counties. This suggests that the population in Foard County may have unique characteristics (possibly age distribution) contributing to higher cancer rates that need targeted interventions.
- Additional Information Needed: To better compare these three counties in terms of cancer incidence, additional information could be helpful:
- Age Distribution: Since age-adjusted rates are provided, knowing the age distribution of each county’s population would be important. This information would aid in understanding if the differences in rates are due to age-related factors.
- Risk Factors: Information on common risk factors (e.g., smoking, diet, genetics) prevalent in each county could help explain variations in cancer rates.
- Access to Healthcare: Availability and quality of healthcare services in each county could influence cancer detection and reporting rates.
- Cancer Types: Knowing the types of cancers prevalent in each county could provide insights into which cancers are contributing most to the incidence rates.
In conclusion, Harris County’s data is likely the most reliable due to its larger population size. Foard County requires the most preventative intervention based on its high crude cancer rate. Additional information on age distribution, risk factors, healthcare access, and cancer types would be valuable for a comprehensive comparison of these counties’ cancer incidence rates.
QUESTION
Description
- g the lists below, write interpreting the data. In your interpretation, answer the following questions:Which County data would you consider the most reliable? Why? What about the least reliable data, and why?
- Which County needs the most preventative intervention as it pertains to cancer incidence rates? Why?
- What additional information or additional data do you think you would need to best compare these three counties in terms of cancer incidence, if any?Harris County
- Population at risk – 22237304
- Cases – 77228
- Crude rate – 347.3
- Age-adjusted rate – 400.1
- 95% CI – 397.2
- 95% CI – 403.1
Bell County - Population at risk – 1659684
- Cases – 6733
- Crude rate – 405.7
- Age-adjusted rate – 480.8
- 95% CI – 469.1
- 95% CI – 492.6
Foard County - Population at risk – 6315
- Cases – 42
- Crude Rate – 665.1
- Age-adjusted rate – 404.9
- 95% CI – 280.4
- 95% CI – 573.7
Understanding epidemiological data, even if you don’t develop the data yourself, is important for putting together health campaigns and designing interventions. The data convinces decision makers that these efforts are necessary to begin with. Let’s delve a little bit into the data terminology.
Morbidity
Morbidity, simply put, is a situation in which an individual’s health and well-being are in a state that is not considered normal. In other words, morbidity is the disease state of an individual. In epidemiology, we deal with population rather than an individual. Therefore, morbidity can also be defined as the frequency of disease, illness, injuries, and disabilities in a population.
It is important to examine morbidity because it provides an opportunity to understand the magnitude of population suffering from an illness. It helps us understand how a disease can impact quality of life of an individual. It also provides us quantitative evidence to develop programs to control and prevent a disease. It is important to examine morbidity because it allows policymakers to allocate appropriate resources for control and prevention of a disease. This leads to overall well-being of a society. Morbidity also gives an idea of economic cost of an illness and how we may save money by eliminating or reducing an illness.
The two most commonly used measures of morbidity are incidence and prevalence.
Incidence
Incidence refers to the number of new events or cases that occur in a population. If it measures the rate at which new events or cases occur in a specified population, then it is called the Incidence rate. Incidence rate is a measure of the probability of developing the disease or illness for individuals in the specified population during a given time period. The numerator is the number of new cases only found during a given time period under study. The denominator is the specified population at risk during the time period under study. The denominator may also be person-years of exposure, often used when the length of time subjects are part of study is variable.
Prevalence
Prevalence refers to the number of existing cases, disease, or illness in a specified population at a given point in time. If it measures the proportion of existing cases in a specified population, then it is called the Prevalence rate. In prevalence, numerator includes both new and existing case.
Mortality is the definite end point of life. It is something of interest to understand its causes and factors ways we can delay it. It is important to understand the differences in the dying from various diseases in different populations. Mortality can provide information about the severity of disease and provide us insight into whether treatment over time has become more effective or not.
Mortality rate is the measure of the number or frequency of death in a specified population during a specified time interval. The mortality rate may be crude, specific (category or subgroup like age, sex, ethnicity), or adjusted to a standard population.
An adjusted rate is an artificial summary rate that allows comparison between populations without the influence of a confounding factor (or factors). The rate depends on the standard population used to adjust it.
Crude death rate
Death rate within the entire population from all causes. The denominator is the entire midyear population.
Cause or disease specific death rate
Rate at which deaths occur for a specific cause, The numerator is the number of deaths attributed to a specific cause. The denominator remains the size of the population at the midpoint of the time period. An example of cause or disease specific death rate will be all deaths due to heart disease in the United States.
Age-specific mortality rate
A mortality rate limited to a particular age group. The numerator is the number of deaths in that age group; the denominator is the number of persons in that age group in the population. For example, deaths in 15-24 years age group.
Sex-specific mortality rate
A mortality rate among either males or females. Both numerator and denominator are limited to the one sex.
Race-specific mortality rate
A mortality rate related to a specified racial group. Both numerator and denominator are limited to the specified race.
Rate adjustment is used when two or more populations have different distributions of a demographic variable such as age, sex, and race. It is also used to remove the effect of differences in age or other confounding variables. One of the most important purposes of rate adjustment is to adjust for known confounding factors such as age, sex, race/ethnicity, social class, smoking status, birth weight, maternal age, and so on.
Age is the most commonly adjusted factor. Adjusted rates are not real because they apply actual specific rates to a hypothetical standard population. Meaningful age groups should be used for adjustment of age; usually 3 or more groups are used. The U.S. population in 2000 is generally used as a standard population. An example of a typical age-adjusted rate is cancer incidence and mortality rates. Cancer in children is defined differently than in adults, so children should not be included in cancer rate calculations when talking about adults cancers so the rate is age-adjusted to remove children.
Advantages Disadvantages
Frequency (Raw Count)
Advantages – Actual number of events
Disadvantages – Influenced by the size of the population
Crude rates
Advantages – Rate without any adjustment Easy to calculate
Disadvantages – Influenced by population characteristics (e.g., age, race)
Specific rate
Advantages – Calculated for homogenous subgroups Useful in identifying subgroups at risk can be used to Disadvantages – compare populations Cumbersome when comparing more than two subgroups of two or more populations
Adjusted Rate
Advantages – Differences in adjusted factor between population removed Permits unbiased comparison relative to adjusted factor Allows for comparing different populations
Disadvantages – Artificial rate Adjusted rates do not represent risk of dying in the population
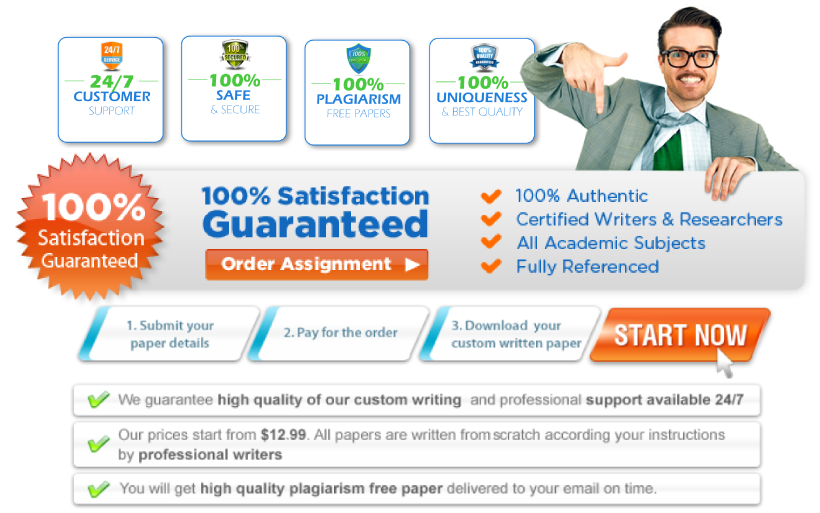