Data Mining Software of Orange Discussion Questions
ANSWER
Python Part:
- Build a Neural Network Classifier: You will need to create a neural network classifier in Python that takes the 3 hidden unit outputs from the autoencoder as inputs and classifies them into one of eight classes. You can use libraries like TensorFlow/Keras or PyTorch to build and train the classifier. Here’s a basic example using TensorFlow/Keras:
import numpy as np
import tensorflow as tf
from sklearn.model_selection import train_test_split
from sklearn.metrics import accuracy_score, confusion_matrix
# Load the hidden layer outputs (hx) and labels
hx = np.load("hidden_outputs.npy")
labels = np.load("labels.npy")
# Split data into training and testing sets
x_train, x_test, y_train, y_test = train_test_split(hx, labels, test_size=0.2, random_state=42)
# Build a simple neural network classifier
model = tf.keras.Sequential([
tf.keras.layers.Dense(32, activation='relu', input_shape=(3,)),
tf.keras.layers.Dense(8, activation='softmax')
])
# Compile the model
model.compile(optimizer='adam',
loss='sparse_categorical_crossentropy',
metrics=['accuracy'])
# Train the model
model.fit(x_train, y_train, epochs=10, batch_size=32)
# Evaluate the model on test data
predictions = model.predict(x_test)
rounded_predictions = np.argmax(predictions, axis=1)
# Calculate accuracy and confusion matrix
accuracy = accuracy_score(y_test, rounded_predictions)
conf_matrix = confusion_matrix(y_test, rounded_predictions)
print("Accuracy:", accuracy)
print("Confusion Matrix:\n", conf_matrix)
Replace “hidden_outputs.npy” and “labels.npy” with the actual file names for your hidden layer outputs and labels.
- Report Results: Report the raw accuracy and confusion matrix results from the classifier.
Weka Part:
- Transfer Hidden Layer Outputs to ARFF File: You’ll need to save the hidden layer outputs as an ARFF file. You can use Python to generate the ARFF file as follows:
import numpy as np
# Load the hidden layer outputs (hx)
hx = np.load("hidden_outputs.npy")
# Create an ARFF file
with open("hidden_outputs.arff", "w") as f:
# Write ARFF header
f.write("@RELATION hidden_outputs\n")
for i in range(3):
f.write(f"@ATTRIBUTE f{i+1} REAL\n")
f.write("@ATTRIBUTE class {p1,p2,p3,p4,p5,p6,p7,p8}\n")
f.write("@DATA\n")
# Write data
for i, row in enumerate(hx):
class_label = labels[i]
f.write(f"{row[0]}, {row[1]}, {row[2]}, {class_label}\n")
Replace “hidden_outputs.npy” with the actual file name for your hidden layer outputs.
- Load ARFF in Weka and Use J48: Open Weka, load the “hidden_outputs.arff” file, and use the J48 classifier to test it on the training data.
- Report Results: Report the accuracy and confusion matrix results from Weka.
Quality of Extracted Features:
To comment on the quality of the extracted features, you can analyze the performance of both the Python neural network classifier and the Weka J48 classifier. If both classifiers achieve high accuracy, it indicates that the extracted features from the autoencoder’s hidden layer are informative and useful for classification. If the accuracy is low, it suggests that the features may not be very discriminative for the given task. You can also inspect the confusion matrix to understand how well the features are distinguishing between different classes.
QUESTION
Description
Python Part
You are given code that builds an 8-3-8 autoencoder in ( file attached below ) developed in Google Colab in files. There is also code to extract the outputs of the hidden layer. So, if this was learned well the outputs of the 3 hidden units for the 8 examples will enable you to build a classifier that uses those outputs as inputs and correctly classifies them.
1- You must build the (at least single layer) neural network that does this and show the results. The results will be the output of the predictions of the new network. Report the raw values and the values rounded to the nearest integer (i.e. 0 or 1).
Weka Part
You must take the outputs of the original hidden layer and transfer them to an arff file. Then load that into Weka. Your arff header will be something like that below (I called the outputs px for each of the places a 1 could be).
@ATTRIBUTE f1 REAL
@ATTRIBUTE f2 REAL
@ATTRIBUTE f3 REAL
@ATTRIBUTE class {p1,p2,p3,p4,p5,p6,p7,p8}
Use J48 to test on training data.
1- Show the result in terms of accuracy and confusion matrix.
2- Comment on the quality of the extracted features.
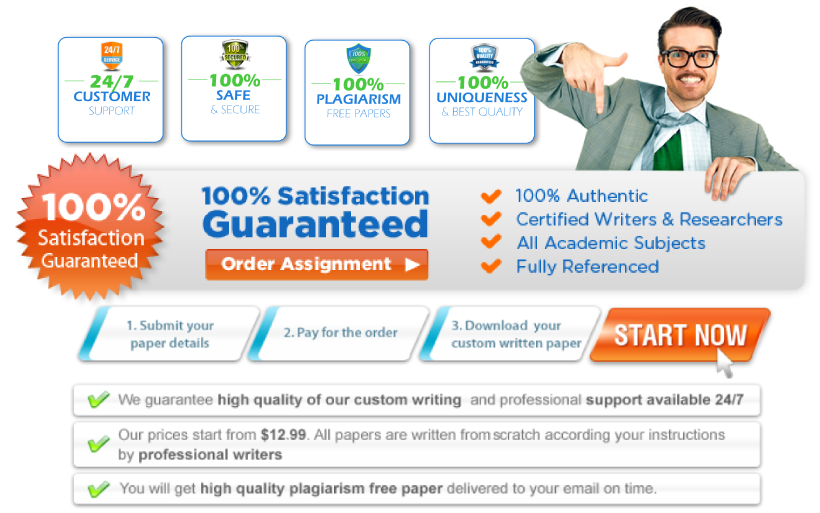